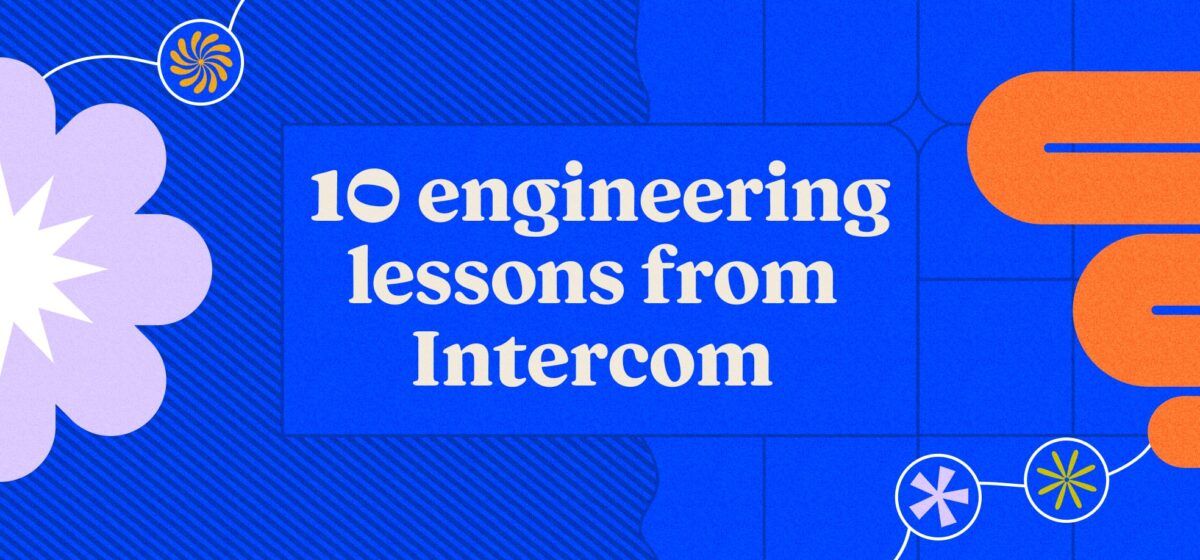
10 engineering lessons from 6 years at Intercom
Intercom has a unique engineering and product culture – discover the main lessons around product and technology learned by one of our engineering directors during his time here.
If shipping is your heartbeat, you're in the right place
Intercom has a unique engineering and product culture – discover the main lessons around product and technology learned by one of our engineering directors during his time here.
AI & Automation 20 min read
ChatGPT has revolutionized the world’s perception of artificial intelligence – but how did we get here exactly? Explore the remarkable advancements and milestones in natural language processing that led to this groundbreaking innovation.
Engineering 5 min read
Without rock solid availability, nothing else matters. That’s why we have been continually investing in our people, systems, and processes to maintain Intercom’s high standards of availability.
Engineering 5 min read
We have truly global ambitions to bring our mission of making internet business personal to the biggest enterprise customers across the world. That means ensuring reliability by default, and the ability to accommodate massive scale as we grow.
Engineering 2 min read
Intercom is supporting the Embroider Initiative to build Ember’s next-generation build system – find out how and why we’re involved in this open-source software project.
Product & Design 5 min read
Our engineering principle “shape the solution” allows us to deliver better customer value and maintain a team of highly engaged, aligned and motivated individuals. Shaping the solution means that we never blindly execute on requirements defined by others. We deeply understand the value of our work, and help design solutions which efficiently deliver that value.